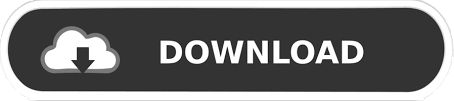

9.3.4 Use of Fractal Dimension Estimators for Texture Analysis.9.3 Quantifying the Microarchitecture of Trabecular Bone.8.5.3 Separating Adjacent Bacteria Under Phase Contrast Microscopy.8.5.2 Using Linear Features to Quantify Astrocyte Morphology.8.5.1 Neurite Tracing for Drug Discovery and Functional Genomics.8.4.3 Linear Feature Detection and Analysis Results.8.3.5 Results for GPU Linear Feature Detection.8.3.2 Linear Feature Detection Performance Analysis.8.3.1 Overview of GPUs and Execution Models.8.2.3 Finding Features Next to Each Other.8.2.2 Check Intensities Within 1D Window.8.2.1 Linear Feature Detection by MDNMS.7.5.3 Nerve Fiber Detection Comparison Results.7.5 Quantitative Analysis and Evaluation of Linear Structure Detection Methods.7.4.4 Postprocessing the Enhanced-Contrast Image.7.4.3 Separation of Nerve Fiber and Background Responses.7.4.2 Local Orientation and Parameter Estimation.7.4.1 Foreground and Background Adaptive Models.7.3.2 CCM Image Characteristics and Noise Artifacts.7.3.1 CCM for Imaging Diabetic Peripheral Neuropathy.


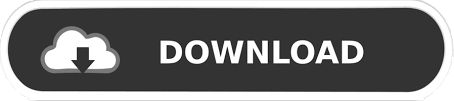